In today’s data-driven world, organizations need to manage vast amounts of data efficiently while deriving meaningful insights. Traditional data architectures often face challenges, such as the need to separate raw data (data lakes) from processed data (data warehouses). The lakehouse concept combines the best of both worlds—offering the scalability and flexibility of a data lake with the analytical power of a data warehouse.
Table of Contents:
- What is a Lakehouse?
- Key Features of a Lakehouse
- Benefits of a Lakehouse
- Popular Lakehouse Platforms
- Challenges and Limitations
- The Future of Lakehouses
- Conclusion
What is a Lakehouse?
A lakehouse is a unified data architecture that brings together the strengths of data lakes and data warehouses. It allows organizations to store raw, unstructured data alongside structured, processed data in one platform, providing a comprehensive solution for data storage, management, and analytics. By bridging the gap between data lakes (which store vast amounts of raw data) and data warehouses (which manage structured, queryable data), the lakehouse offers a flexible and scalable approach to data analytics.
Unlike traditional data lakes, which often lack the structure necessary for high-performance analytics, a lakehouse introduces features like schema enforcement, data governance, and transactional support (ACID properties). This makes it possible to run complex analytics on both raw and processed data without sacrificing data quality or consistency.
Key Features of a Lakehouse
- Unified Data Management: Lakehouses combine structured, semi-structured, and unstructured data into one platform, enabling businesses to store all types of data without needing separate systems.
- ACID Transactions: Ensures data consistency and integrity, allowing users to run complex analytics with confidence in the quality and reliability of the data.
- Schema Enforcement: Supports structured data while still allowing the flexibility to store raw, unprocessed data, making it easier to manage diverse data types.
- Scalability: Built on cloud infrastructure, lakehouses provide elastic storage and compute resources that can easily scale as data grows.
- Real-Time Analytics: Lakehouses are designed to support both batch and real-time data processing, empowering organizations to access insights immediately.
Benefits of a Lakehouse
- Simplified Data Management: Reduces the complexity of managing separate data lakes and warehouses, offering a more integrated and efficient approach to data storage and analytics.
- Cost-Effective: By combining data lakes and warehouses, businesses can eliminate the need for multiple systems and reduce operational costs.
- Faster Decision-Making: Real-time and batch processing capabilities enable quicker insights, which helps businesses stay agile and competitive.
- Advanced Analytics: The ability to store both raw and processed data in the same environment allows organizations to apply machine learning and AI models directly, enhancing their analytics capabilities.
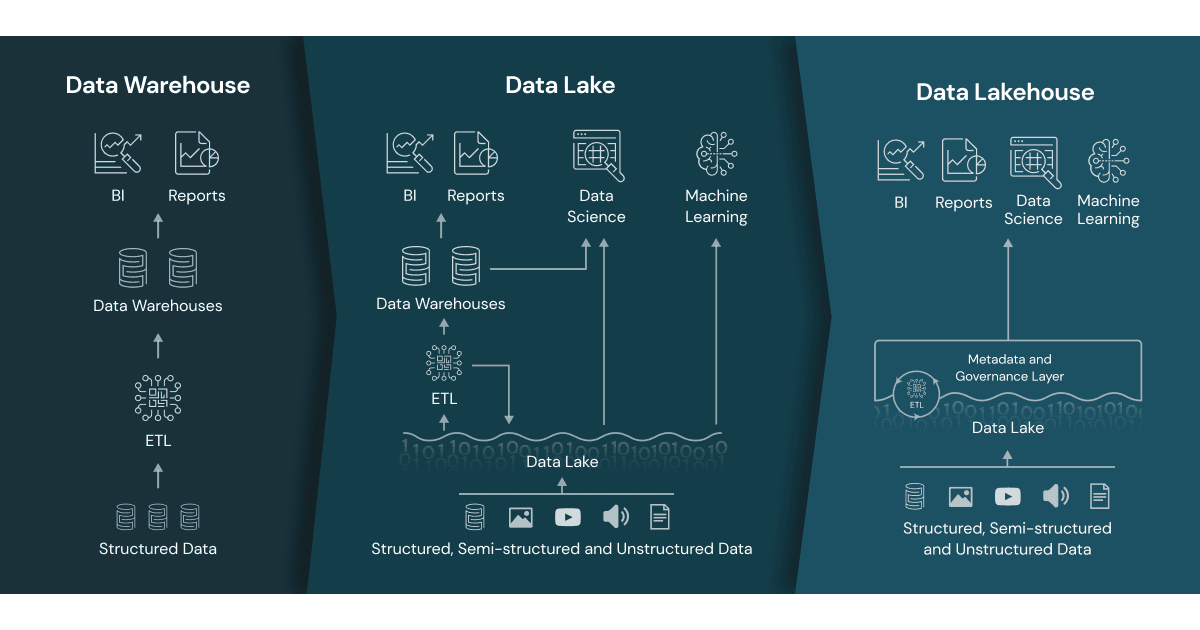
Popular Lakehouse Platforms
Several platforms provide lakehouse solutions, including:
- Databricks: Known for its powerful integration with Apache Spark and Delta Lake, Databricks enables real-time data processing and collaborative analytics.
- Google BigLake: A unified data architecture that allows businesses to manage and analyze both structured and unstructured data across Google Cloud.
- AWS Lake Formation: A managed service from Amazon that simplifies the process of building and managing data lakes with built-in security and governance features.
- Snowflake: A cloud-based platform that blends data lakes and warehouses, enabling seamless data sharing and advanced analytics.
Challenges and Limitations
Despite its advantages, the lakehouse architecture comes with challenges:
- Data Governance: Managing data quality and ensuring consistent, accurate data across a unified system can be complex, particularly with large, diverse datasets.
- Performance: Ensuring fast query performance on large datasets can be challenging, especially when dealing with raw, unstructured data.
- Skill Gaps: Building and maintaining a lakehouse requires specialized knowledge in data engineering, machine learning, and cloud technologies, which may be in short supply.
The Future of Lakehouses
As technology continues to evolve, lakehouses will see advancements in real-time data processing, integration with AI/ML tools, multi-cloud capabilities, and improved user interfaces for easier access to analytics. With these developments, lakehouses will continue to be a critical solution for organizations looking to manage large volumes of diverse data and make data-driven decisions faster.
Conclusion
The lakehouse concept is revolutionizing data architecture by providing a scalable, flexible, and integrated solution for managing diverse datasets. By combining the strengths of data lakes and data warehouses, lakehouses enable businesses to gain actionable insights, drive innovation, and make data-driven decisions. Despite challenges, the future of lakehouses looks promising with advancements in AI, real-time analytics, and multi-cloud integration, positioning them as a key element in modern data strategies.